Abstract
The COVID19 outbreak, which started in Wuhan, is now spread across 200+ countries with over 6 million reported infections and a death toll over 350 thousand. In response, and primarily in the absence of a vaccine, many countries have implemented lockdown to ensure social distancing and started rigorously quarantining the infected subjects. In this study, we attempt to identify the most potent component(s) in the system that can be manipulated via human intervention. Firstly, analysis of the metadata for 93 countries showed a reduction in the estimated reproduction number (a month post-infection) is correlated to the testing rate in a country. To systematically study the dynamics of infection we next built epidemic models for 23 different countries and calibrated the confirmed, recovered, and dead population trajectories in the model to the respective data from WHO. The countries chosen either had the infection peak long crossed; peak recently reached but still with significant daily infection, or, infection peak is yet to arrive. Our model successfully fits data from all 23 countries and provides us with incubation time, transmission rate, quarantine, recovery, and death rates for each country. With further analysis, we found infection spread towards a much larger second wave can be controlled via a rigorous increase in the quarantine rates that, we show, can be tailored in a country-specific manner; for instance, we found the USA or Spain might require a 10 fold increase in testing/ quarantine rates compared to India to control the second wave post lockdown. Our data-driven modeling and analysis thus pave a way to understand and manipulate the infection dynamics during and post lockdown phases in various countries. The findings can also be used to strategize the testing and quarantine processes to manipulate the spread of the disease in the future.
Introduction
Declaration of the coronavirus pandemic by WHO severely overhauled global economic and social endeavors for last few weeks [1]. With the first case encountered in Wuhan, China, in November 2019 and subsequent outbreak in Hubei province, the virus now has spread to more than 200 countries globally. Western Europe and the United states are severely affected with more than 4.5 million infected population and global death toll rising over 300 thousands [1]. Many European countries already started imposing strong mitigation measures through nationwide lock-down in order to maintain effective social distancing within the population. Even with nationwide lock-down, many countries are struggling to contain growth of the infection[2]. Hospitals are getting overwhelmed with patients and are running out of necessary equipment and medicines [3,4]. The health-care personnel were in severe crisis of medicines, masks, testing kits, ventilators etc. The vaccine preparation is already on it’s way at a breakneck pace[5] but a fully operational vaccine after clinical trial is expected to take at-least a year from now. Until then, isolating the infected population by aggressive testing and maintaining strong social distancing measures are adopted as the two most effective ways to deal with the current situation [6]. From the experience in Wuhan, we learned that the outbreak can be effectively contained with strong social distancing measures [2,7]. On the other hand Singapore, Hong Kong and South Korea took a different strategy by aggressive testing and isolating the infected population without imposing nationwide lock-down [6]. However, at this moment both the strategies are argued as equally essential to deal with the situation, especially in Europe, US and countries with high population density like India. Thus In this global emergency scenario, and in the absence of vaccines, model driven strategies to contain the infection rate could be of immense use. Hence, in general, if we can predict the spread of the infection and project possible numbers as well as estimate the social and medical factors influencing the spread of the disease, it would help policy makers in considering multiple strategies to address the state of infection that would also have far reaching socio-economic implications. There indeed is an upsurge in epidemic models to predict possible projections of the current situation in different countries [8-11] which aims to help the policy makers and the medical practitioners to prepare for upcoming situations. Along with prediction, analysis of the models should also inform us with possible quantitative measures to deal with the current and subsequent waves of the infection.
In this paper, we present a dynamic epidemic model for the spread of coronavirus. By quantitatively calibrating the time series data(Data from WHO [1]) for confirmed, recovered and dead population for 23 different countries with various stages of infection, we made an estimate of different important parameters like incubation time, transmission rate, rate of quarantine, recovery and death rate, that controls the infection dynamics in a given country. We introduced lock-down in our model to observe the effect of social distancing and also estimated the effectiveness of implementation of lock down in individual countries. Using the best fit parameter sets, a prediction of the infected numbers for different countries has been projected. Variations in the reproduction number as well as variation in reduction of reproduction number with time is also observed to be correlated with different demographics and socioeconomic quantities, including health-care facilities, which we show by building statistical regression models. The key insights from our study are 1. lock-down start time following infection is the most sensitive parameter determining the final infection status w.r.t the first wave of infection 2. At any stage of infection, rigorous and country specific tailoring of testing and quarantining can accelerate the time to peak and should eventually contain the infection 3. Lock-down removal would most likely start the second wave irrespective of the time of early lockdown to contain the first wave. This is typically because even in countries with a minimal number of asymptotic infected people, imported or local, the susceptible subjects can get exposed and infected eventually. Analysis of our model quantitatively shows, the most effective way to rapidly contain the second wave of infection would be immediate early lock-down and rigorous testing coupled to systematic quarantining, which could reduce the total time of lockdown and size of infected population in a country.
Results
Doubling rate of the infection is determined by the testing rate
In order to explore the dynamics of the COVID19 infection spread, we took the daily confirmed infection time course data from WHO and clustered the region-wise data according to their dynamic pattern. A hierarchical clustering algorithm (hierarchical clustering from pheatmap package in R) is used to analyze the dynamics of 93 countries selected (each country with at least 1000 infections per day). The provinces in China clearly are clustered together since infection spread happened at the earliest times. Then the infection spreads to different parts of Western Europe and the United states. The number of new cases in Western Europe and the United states are much higher compared to other parts of the world which can also be seen as close proximity of these countries in the clustering analysis (Figure 1A, color bar represents log transformed value of the daily confirmed cases). Next, we calculated the doubling rate from the time series of the countries. The doubling rate is defined as the inverse of the doubling time, i.e., how much time does the population take to double the number of infections (Figure 1B). The clustering analysis shows similar patterns, although, there are large region to region variations in the doubling rate. Using a stochastic nearest neighbor algorithm (tSNE), we projected the 93 dimensional time-course data onto two dimensions (Figure 1C). tSNE is a machine learning technique for dimension reduction of high dimensional data [12] extensively utilized for visualization of high dimensional data in diverse fields such as computer graphics [13], neuroscience [14], medicine [15] to protein structure[16] or embryonic development[17]. The analysis provides us with clearer visual information about different countries and their proximities according to their respective infection trajectories. Here also, we observed that the provinces in China are very closely placed and countries in Europe form a different cluster. Similar analysis was conducted for the doubling rate time courses(Figure 1D). An estimate of reproduction number is calculated from the doubling rate rate using an incubation time of 4 days. We observed a 20% CV in the reproduction number among countries. These results indicate that there is a substantial country-wise variability in the infection spread. These variations may stem from each region’s different demography, health-care facility, general health or factors implicit to a given country.
The Covid-19 outbreak in different countries demonstrates large variabilities in the dynamics. (A) The heat map displays the clustered dynamics for 93 different countries for the daily cases, shown in log scale. (B) The heat map represents the doubling rate as a function of time and clustered it according to the dynamics of the doubling rate. The results are shown in exponential scale (C) The tSNE plot based on the daily cases dynamics (D) The tSNE plot based on the doubling rate dynamics (E) The scatter plot shows the relation between the test/1000 and the doubling rate at the start of the outbreak and (F) after 30 days of the outbreak.
We now extracted the doubling rate at the start of the infection and after 30 days. Although, the initial doubling rate does not show any correlation with any of the factors,(Figure 1E) the doubling rate at 30 days exhibits significant correlation with number of tests per million (Figure 1F). This indicates the possibility that countries performing more tests on the population are able to manage the infection rate much more efficiently. The total population size also displays significant correlation. This could be due to the fact that countries with higher population size usually tend to have lower test per million rate. In order to estimate different parameters controlling the infection dynamics in different countries we next constructed dynamic epidemiological models for multiple countries and.
A compartmental epidemic model with distinct infection (I) and quarantined (Q) compartments captures confirmed, recovered and dead trajectories simultaneously
From the group of 93 countries, we selected 23 countries, comprising a combination of early, mid and late stage of infection, and fitted their confirmed (Co), recovered(Re) and dead(De) population trajectories (Methods for details). The standard susceptible, infected, recovered (SIR), or SEIR(E = exposed) model did not simultaneously fit the trajectories Co/Re/De in most of the countries (data not shown), but, Implementing a quarantine compartment (Q), which provided a time delay between infected and removed compartments, dramatically improved the fit quality. The Q compartment also ensured a distinction between infected and identified (Q) and infected but unidentified(I) subjected in a population. The SEIQR model divides the population in five compartments namely susceptible (S), exposed (E), Infected (I), Quarantined (Q) and removed (R), which contain both recovered and dead population [12]. Figure 2A shows the structure of the SEIQR model. Here a susceptible person can be exposed to the infection through transmission from an infected person. After exposure, the symptoms are exhibited within an incubation time and the infected individual either recovers or dies after a time, represented by a recovery or death rate. Figure 2C shows cartoon trajectories of confirmed, recovered and dead population which depicts the key qualitative feature - confirmed > recovered > dead. Dynamics of the system are captured by a set of 6 coupled ordinary differential equations (details in methods). The calibration data for each country comprises the time courses of the number of confirmed, recovered and dead people. Through model fitting we estimated the parameters that best explains the Co/Re/De trajectories simultaneously of each country. Model fitting also includes a lockdown function (Figure 2B). The lock down is introduced in the model through a reduction in the transmission rate that follows an inverse sigmoid function. The process of lockdown is controlled by three variables-time of lockdown (start time of lockdown implementation), strength of lockdown (the extent of lockdown in a country, 0.1 would mean 90% lockdown implemented) and the effectiveness of lockdown (how fast the maximum lockdown is achieved from the lockdown starting time point); during the calibration these parameters were also estimated for each country.
The description of the SEIQR model: The model utilized to fit the cumulative confirmed, recovered and dead cases comprises susceptible, exposed, infected, quarantined, recovered and dead compartments. The lockdown is implemented through a sigmoid function as indicated. The quarantined, recovered and dead cases together comprise the confirmed cases.
Figure 3 shows the model fits the confirmed, recovered and dead populations of 8 representative countries with infection size varying from almost 1.5 million (USA) to just above a thousand (Iceland). The Co/Re/De fits to all the 23 countries are shown in supplementary figure S1, S2 and S3, respectively. Here we fit the cumulative trajectories for Co/Re/De simultaneously. The confirmed trajectory by definition comprises [quarantined + recovered + dead] population and the infection(I) compartment represents the unidentified/unknown infection cases present in the population. The assumption is rooted in the fact that once an infected person is tested positive he/she will be quarantined, thus, only the infected but untested subjects can further infect the susceptible.
The SEIQR models fit the data for 8 countries as indicated for the cumulative confirmed, recovery and death cases. The figures indicate the cumulative data and the corresponding fit based on the SEIQR model.
Next we extracted the daily confirmed cases from the fitted cumulative trajectories from the model and compared it with data. Figure 4 shows daily confirmed trajectories from the 23 countries. The model successfully reproduces daily trajectories from 1. saturating countries where the first wave of infection is almost over (Australia, Austria, Iceland, France etc,) 2. The countries reaching a peak of infection but still significant daily infections are observed (USA, UK) 3. The countries where the peak is yet to arrive (India, Russia, South Africa). Also, on occasions, such as for Japan, although the fit to cumulative trajectory is reasonably good, the daily trajectory matches are not good, this may indicate influence of factors (in countries like Japan) that are not yet incorporated in our simplified model. To this end we obtain the parameters controlling various steps of the infection process. For instance, the incubation time, which is the time interval between the exposure to the virus and display of symptoms, typically varies between 2 to 14 days [19]. Our model fits show a mean incubation time of 8.1 days. However, there are also countries where incubation time appears much higher, eg. India (23 days), suggesting the presence of a large number of asymptotic populations. Further, we also observed a large variability in effective lockdown among countries (CV=126%), suggesting plausible influence of different social structures within countries in the implementation. This may result from multiple implicit factors specific to a country such as socio-economic structure, state healthcare, population size or other similar factors.
The fitted model also reproduces the daily cases. The daily infected cases were calculated from the fitted confirmed trajectories and the data was calculated from the respective cumulative data trajectories of the respective countries.
The medical infrastructure and testing rate may influence the infection spread rate
As observed from the model, the infection rates substantially vary from country to country. Here, we ask the pertinent question why the infection rate and it’s reduction vary from one country to another. The socio-economic factors like health infrastructure, demography or population size may influence the infection rate depending on how quickly and efficiently a country’s health care facilities react to emergencies. Similarly, the reaction can also be limited by the country’s population density or demography with respect to age distribution, as it is in general expected that the younger population would be able to react to a new infection more efficiently compared to the older population. In fact, the death rate indeed is substantially high for older people with COVID19 infection [21].
Here, in order to explore social, economic and demographic factors which may influence infection rate of the model, we extracted medical infrastructure, health care spending, demography and population density datasets for all the 23 countries and a correlation analysis based on linear correlation were performed. Using the fitted trajectories from the model, R0 values were first calculated (Methods) over time for all the countries (Figure 5A) and the initial R0, R0 after 30 days (R30) were extracted from the time course. Correlation analysis of R0, R30 with the health care and demographic factors were performed separately. Although R0 was not found to be correlated with any of the parameters, R30 values show significant correlation with doctors/100, life expectancy and test per million (Figure 5B). Other factors like hospital beds/1000 and health care spending tend to show some negative correlation as noticed from the scatter plot, however, the correlations are not significant due to the relatively small number of samples/country number used for fitting. The initial outbreak although does not depend on the heath-care facility, in absence of early lockdown, how effectively the countries would react to contain the reproduction number, can be correlated to their health infrastructure. However, importantly, the pandemic has affected almost all the countries in the world, irrespective of their healthcare or socio-economic structure, and the need of the moment is to devise scientifically informed strategies to manipulate the spread of infection and minimize the loss of human life which can perhaps be adopted by most countries tailored to their infection status. In an attempt to look for such general strategies to circumvent the infection we systematically explored the calibrated SEIQR models further.
The infection rate correlates with testing rate and the medical infrastructure. (A) The reproduction number is calculated from the fitted model trajectories and were plotted as a heat map. The results are shown in linear scale (B) The scatter plot for the demographic and medical factors as a function of reproduction number for all the 23 countries (C) The statistical significance of the Pearson correlation between the reproduction number and the parameter indicated.
Extent of testing and quarantining can critically determine the infection size and it’s duration in a country
To understand the relative sensitivity of the model parameters such as β (Susceptible → Exposed), α1 (Exposed → Infected), α2 (Infected → Quarantined), γr (Quarantined -> Recovered) γd (Quarantined -> Dead), as well as, the parameters controlling the lockdown implementation function in a given country (Start time, implementation strength and effectiveness of lockdown) we next carried out a sensitivity analysis by perturbing each parameter and capturing the effect of such perturbation on the peak position, maximum amplitude and area under curve(AUC) for a country and a cumulative sensitivity score is derived for such variation. The local sensitivity plot (Figure S4) shows the effect of such perturbations in the 23 countries. The analysis pointed us to the parameters significantly controlling the infection dynamics (sensitivity of the confirmed trajectory is shown in figure S4) in different countries. Out of the most sensitive parameters, β can not be altered when lock down is removed and α1 is not directly tunable via human intervention. Only the ‘time of lockdown’ and α2 are important from an implementation perspective. With respect to the first wave of infection the implementation window for the ‘time of lockdown’ is already missed, but α2, although not the most sensitive among all parameters can still be tuned anytime by changing the testing and quarantine strategies. Notably, the effect of α2 variation (in the range chosen for sensitivity analysis, [0.75-1.25]* α2 Fitted) has diverse sensitivity profile in the group of countries; countries like South Korea is extremely sensitive to α2 variation where it is known that the testing and quarantining is one of the most rigorous. To understand how the manipulation of α2 might change the long term infection dynamics in the present scenario, we next simulated the models for 300 days, with lock down. From the simulated time course (Figure 6A,B, shown for India and Korea, two representative countries with high differences in quarantine rate and time to infection peak positions), peak position from the start of the infection, the shift between undetected infection(I) peak and the tested and ‘confirmed’ peak were estimated for the 23 countries. Our analysis showed peak position (Tp) is negatively correlated with the quarantine rate, indicating that the more quarantine facilitates an earlier peak (Figure 6C). This suggests the infection time can be manipulated by quarantining more people. The peak shift(Ts), or the time between the confirmed and undetected infected peak position, is also smaller if the quarantine rate is high, indicated by the negative correlation between Ts and quarantine rate (Figure 6,D). Subsequently, the fraction of undetected infection is also low if the quarantine rate is high for a given country(Figure 6E). These results suggest that by quarantining a lot of infected people through testing, the infection dynamics and time to peak can be closely predicted with lesser error. Additionally, this may lead to faster exit from the first wave of infection, as seen in countries like Korea. We also observed correlation of Tp, Ts and fraction of undetected infection with the incubation rates (Figure 6E, F, G). This is due to the correlation between incubation and quarantine rates in the calibrated models.
The quarantine rate influences the arrival of the peak and the fraction of undetected infection. (A) The simulated infected and quarantine cases for two representative countries with a low(India) and (B) high quarantine rate. (C)The confirmed cases peak position as a function of the the quarantine rate (D) Peak shift between the undetected infection(I in figure 2A) and the confirmed cases as a function of quarantine rate (E) The percentage of undetected infection cases as a function quarantine rate (F)The peak position, (G)peak shift and (H)percentage of undetected infection as functions of effect of incubation rate.
Optimum quarantine rate change, uniquely for each country, can effectively reduce the infection post lockdown
The modeling and analysis so far considered a scenario when most of the countries are in a lock-down state. However, how the infection dynamics would evolve post lock down, or even during lockdown (for countries yet to reach a peak), is not clearly understood. Hence, going forward, how to successfully contain the infection is one of the major challenges right now. A recent study suggested a periodic lock down measure to optimize the infection spread and economic activity [20]. Here, using our calibrated models for 23 countries, we next explored how changes in quarantine rate can alter the infection dynamics during and post lockdown phases. Figure7 shows the dynamics of the confirmed cases for Sapin, USA and India, three representative countries with different stages of infection wrt to the first wave. With lockdown continued, changes in α2 accelerates the position to peak, and exits the infection phase faster (Figure 7B-C), but, Spain where the first wave is almost over, increasing α2 doesn’t change the infection profile significantly and even for a five fold increase in testing and quarantine rate the trajectory remains practically unchanged(Figure 7A). However, as the lockdown is lifted, 5 fold increase in α2 (Sapin, USA) appears not sufficient to contain the infection and a much larger 2nd wave can emerge if testing rate is ramped up only 5 fold(Figure 7D-E); India, on the other hand doesn’t show dramatic changes in total number of infection post lockdown with the same increase in α2 value (Figure 7F). We also explored how the total susceptible population size (absolute number of succestable subjects in a population is typically unknown in reality, hence, here for each country the susceptible population size is determined by fitting a population size around the total number of tests carried out in that country) might affect the observed result. Figure S5-S7 shows that the peak of daily confirmed cases for a country and its time to exit from the first wave can robustly be captured in the models for a range of susceptible population size (tested for [0.2-5 fold] of fitted population size).
The effect of lock down removal in India, Spain and the USA. The simulation trajectories for daily confirmed cases are shown for three countries and 4 different quarantine rates with lockdown and lockdown removal, as indicated. The quarantine rates are increased on the 115th day (after 22nd Jan, 2020, the first respored day of infection in Wuhan) and lockdown removal on the same day is considered for these simulations..
Typically, when lock down is removed, the transmission rate (β) would increase substantially. The long term simulation for India, USA and Spain predicted various features of their confirmed time series by the end of June, for varying degrees of quarantine rate, with or without lockdown. For instance, for India the expected number of confirmed cases are estimated to be 450 thousands by the end of June when the lockdown continues and 100 thousands more cases will be added if lock down is removed (Figure 8A, B).However, for both Spain and the USA a substantial change in total confirmed cases could be observed (Figure 8 A,B). The daily confirmed cases show a similar trend for both USA and Spain, but for India, the post lockdown changes in daily cases are relatively less (Figure 8C). This indicates different countries may have different infection spread dynamics after the lockdown is lifted. As seen in figure 8D, a 5 fold increase in quarantine dramatically reduces the fraction of undetected infections in the (model)population, both pre and post lockdown, for all the countries. The increased quarantine rates can facilitate the arrival of the peak a bit earlier for countries like India where peak is yet to arrive, especially, when the lock down is removed(Figure 8E). We also calculated the R0 value just after the lock down is removed. For these 3 countries, an increase in the value of R0 is observed as the lockdown is lifted but the R0 value can be substantially suppressed by five fold increase in the quarantine rate for India. where the 10% increase in R0 due to removal of lock down can be effectively circumvented by 40% reduction in R0 by five fold increase in the quarantine rate (Figure 8F). But, both for Spain and USA, a dramatic increase in R0 is observed which can not be compensated by a five fold increase in the quarantine rate (Figure 8F).
The lock removal effect can be compensated by increased quarantine rate. (A) The increase in accumulated total cases (confirmed infected + unidentified infected) (B) Confirmed cases (C) daily confirmed cases as a function of quarantine rate. (D) The percentage of undetected cases (E) peak and (F) Reproduction number is also shown as a function of quarantine rate
Thus, for Spain and the USA, we explored if much higher quarantine rates would be required to compensate for the enhanced R0 resulting from the lock down release. We performed a set of simulations by systematically increasing the α2 value to 50 times of it’s fitted value for each country. Now, for both Spain and the USA a 50 fold increase in quarantine rate is able to drastically reduce the peak of the second wave (Figure 9). Quantitatively, our simulations suggest that both the USA and Spain require different degrees of α2 change for an effective control of the infection spread (data not shown). Such decisions on fold change increase in testing rate could be optimally derived during country specific implementations, but, we here show 50 fold change in α2 as a representative of a very high change in α2 that can, in general, successfully suppress the spread of infection in such countries. This suggests rigorous testing and quarantining can circumvent the infection even in the countries where the potential 2nd wave could be of many fold magnitude compared to the first infection wave. Further, the time of lockdown removal does not influence the result substantially (data not shown) as lockdown removal in the model does eventually result in a second wave, indicating infection spread can only be contained by optimally increasing the quarantine rate.
Optimal, country specific change in quarantine rate can potentially circumvent the infection spread post lockdown. The simulated trajectories for daily confirmed cases when quarantine rate is increased on 115th day and lockdown is lifted on 130the day for the three countries. Similar results were obtained when both quarantine rate and lockdown removal times are chosen as the same. The three panels depict the current situation, the situation with quarantine rate increased on 115th day in presence of lockdown, and lockdown removed 15 days after changes in the quarantine rates, respectively, as indicated.
These simulations suggest that effective quarantine measures can potentially compensate for the act of lockdown removal irrespective of the time of removal. Based on these results we can argue that delaying the lock down removal only delays the arrival of the second wave providing an opportunity to prepare for testing and quarantine measures, which could be a good strategy. But lockdown alone, irrespective of how early it is implemented, is not enough if it is not complemented by optimal testing and quarantining process. In fact, simulations with an earlier start of the lockdown while keeping the quarantine rate the same did not improve the situation much with respect to the magnitude of the peak of the confirmed cases but it only delayed the arrival of the peak (Figure 10, shown for India, Spain and USA), where such long term lockdown can lead to devastating socio-economic scenarios. Based on our quantitative modeling and analysis, we argue, optimally increasing the quarantine rate is the only effective way to successfully circumvent the amplified transmission both in presence and especially in absence of lockdown.
Earlier lockdown only delays the arrival of the peak but total infected population size remains independent of lockdown start time The daily confirmed trajectories simulated with earlier lockdown time by 0.75 of the current fitted lockdown time and a delayed lockdown time by 1.5 times, is shown, for India, Spain and USA.
Discussion
In this paper, using an epidemic model coupled with statistical regression models we quantitatively explored the time series of infection spread in different countries for the COVID19 outbreak and its relation with quarantine measures as well as medical infrastructure. The reproduction numbers of this pandemic are found to be comparable to the SARS-cov values [22,23] and much higher than the MERS infection[24,25]. We employed the mathematical model and fitted the confirmed, recovered and dead population trajectories from 23 countries where the countries are a combination of early, mid and late stage infections for the first wave of COVID19 infection. The fitted mathematical models display large variabilities in the infection rate among countries as well as the reduction in their infection rates over time, primarily, due to implementation of the social distancing measures. We show that the variabilities can be correlated to some extent by disparate healthcare infrastructure. In the European countries, the infection has spread faster either due to strong airline connection with Wuhan or due to the cold climate but they could control the reproduction number(R0) with time and the first wave of infection is almost over for many such countries (Figure 4). The lockdown measure to implement social distancing which is implemented in almost every country infected with COVID19, is a necessary measure to reduce the infection spread, but how well a country is sampling its population for testing and further how well they quarantine the infected population are also important factors during the lockdown. Lockdown is a preparatory measure for the health care system to reorganize itself to deal with the situation since long term lock down would be detrimental to the economy of any country[26].
In line with the other epidemic models, such as SIR or SEIR models, we assumed a constant initial susceptible population in the fitting process which calculated based on the tests per million conducted in a country. However the actual susceptible population size is unknown and it may be different from the tested population size in either direction. As a result, the number of infected people from the data only captures the infected people out of the tested sample. So to understand the effect of population size on infection peak time and time of completion of the first infection wave, we varied the initial population size in [0.2-5] fold of its fitted population size. Absolute population size does impact the height of peak in each country tested, but the time to peak and the time for completion of the first wave could robustly be captured by varying size of initial susceptible population. Typically the actual infected number of people is expected to be higher than the sampled one’s. This is one of the reasons why increased testing rate is so important in capturing the real magnitude of the infection (and not only the dynamics), in addition to the need of quarantining infectious people to reduce infection spread. However, the projection can provide us with a lower bound on the estimated time to contain the infection so that we can remain prepared.
In conclusion, in developing countries like India with high population density and size, early implementation of lockdown was critical where the delay in lockdown such as in Italy or Spain could have had a much more serious impact due to inadequate health infrastructure. However, reopening the economy is also an impending necessity in many countries under lock down. Thus, to minimize the health hazards of social proximity while being able to continue economic activities will require careful planning and implementation. We propose strategies where rigorous quarantining of the infected subjects is argued as the only effective measure to effectively deal with infection spread post lockdown. As a policy measure, our model suggests that quarantine and testing should be increased substantially after the lockdown is lifted, in order to contain the infection in the coming months. The effective increase in quarantine measure is found to be country specific, depending on the transmission or incubation rates.
In our analysis, we assumed a full lockdown removal. A partial or periodic lockdown removal coupled with increased quarantine rate can also be explored to deal with the situation as studied elsewhere [20]. As there are uncertain components like the number of subjects comprising the susceptible population size in such SIR/SEIR/SEIQR models, we also need to be careful about the possible acceleration in the disease spread due to lockdown removal, as a small unidentified fraction of infected population during the lockdown removal can potentially remain unidentified due to the long incubation period characteristic to this infection. The Spanish flu pandemic in 1918 in fact came back again in a few weeks after the first wave was apparently contained and the second wave was much bigger than the first one [27]. So, even if the first wave of the current corona pandemic is over for many countries, the global population and policy makers need to remain pragmatically careful for possible subsequent waves [9] and should stratize to maximally quarantine the early susceptible population. In the fight against COVID19, it seems critical to act early and act with full force; at the same time, controlling overhyped panic stemming from either political polarization or media misinformation [28] could also be prioritized to bring forward a robust and collaborative global effort to fight the pandemic.
Materials and Methods
The SEIQR model
The model comprises of susceptible(S), Exposed(E), Infected(I), Quarantined(Q), Removed(R, contains two compartments ‘recovered’ and ‘dead’)
The equations are
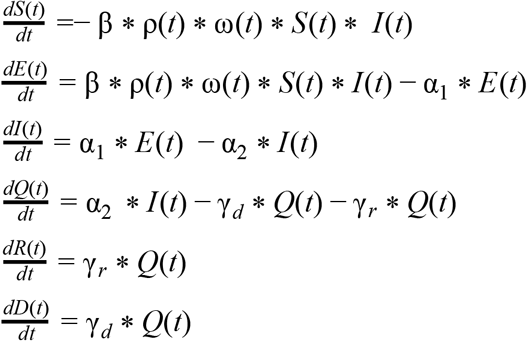
Where S(t), E(t), I(t), Q(t), R(t) and D(t) are the susceptible, exposed, infected, quarantine, recovered and dead population at time t respectively. ρ (t) is the lockdown function and ω (t) is the time when infection starts in a given country after the first day of detection of the infection date is reported in WHO website (22nd Jan, 2020).

ρ (t) varies between 0 and 1(no lockdown) where 0 (full lockdown). ω (t) ensures that the model for a specific country is switched on when the infection begins in that country, hence if the detected case in a country is 40 days after Jan 22nd, the model for that country is switched on 35 days post Jan 22nd (assuming mean incubation time of 5 days), during the calibration.
The lockdown is opened by modifying the ρ (t) function such that ρ (t) returns to 1 from its lockdown status to no-lockdown(1) status in a designated time.
Model calibration
Model calibration involves minimizing an objective function that gives best fit parameter sets for confirmed, recovered and dead populations for a given country simultaneously. We fitted the time series provided by JHU CSSE at github [30] to the SEIQR model developed in the study and minimized the objective function using the lsqnonlin function of MATLAB which minimizes differences in the sum of squares between model and data. To fit the observed cumulative confirmed trajectory for a given country, [quarantined + recovered + dead] from the model is fitted against the confirmed data. The objective function is thus minimized to achieve the best fits for all three trajectory types simultaneously. This was repeated for all the 23 countries independently.
Calculation ofR0and doubling rate
The R0 value is calculated using the R0 package in R [29]. We took the time course data of the daily confirmed cases with a sliding window of 5 days in order to calculate the R0 value locally with time. R0 package takes the time course and the generation time as inputs and generate the R0 value for the time course. The generation time defined as the incubation time (Ti) in this context is extracted from the fitted parameter α1 as . The value of incubation time is generated from a gamma distribution with mean as T1 and a standard deviation of 2.5 in order to perform the calculation of R0.
The doubling rate is calculated at each time point over the trajectory by taking the daily cases at that time and next day. For an exponential growth of the infection, N(t) = N(t + 1)exp(λ) where N(t) depicts the number of infected people at time t
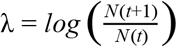
Here t=1 day. The values in the heatmap in Figure 1B are displayed in an exponential scale of doubling rate to avoid negative values. So a doubling rate of 1.4 in exponential scale means the doubling time of 1/log(1.4) = 3 meaning it takes around 3 days to double the number of infected people.
Data Availability
Details of the model simulation and fitting are available upon reasonable request.
Author contribution
US and BG conceived the study. US built the epidemic model, developed fitting and prediction strategies and optimized the parameters. BG performed the regression analysis. US and BG analyzed the results and wrote the manuscript.
Conflict of interest
The authors declare no conflict of interest.
Supplementary Figure legends
Figure S1: The SEIQR models fit the data for 23 countries, as indicated, for the cumulative recovery. The figures indicate the cumulative data and the corresponding fit based on the SEIQR model.
Figure S2: The SEIQR models fit the data for 23 countries, as indicated, for the cumulative recovered. The figures indicate the cumulative data and the corresponding fit based on the SEIQR model.
Figure S3: The SEIQR models fit the data for 23 countries as indicated, for the cumulative dead. The figures indicate the cumulative data and the corresponding fit based on the SEIQR model.
Figure S4: The sensitivity analysis of all the fitted values of the parameters with respect to the change in the trajectories.
Figure S5: The simulated trajectories for lockdown, presence and absence, coupled to varying quarantaine rates, and, with different population sizes, is shown for Spain.
Figure S6: The simulated trajectories for lockdown, presence and absence, coupled to quarantaine rates, and, with different population sizes, is shown for USA.
Figure S7: The simulated trajectories for lockdown, presence and absence, coupled to quarantaine rates, and, with different population sizes, is shown for India.